Network Discovery
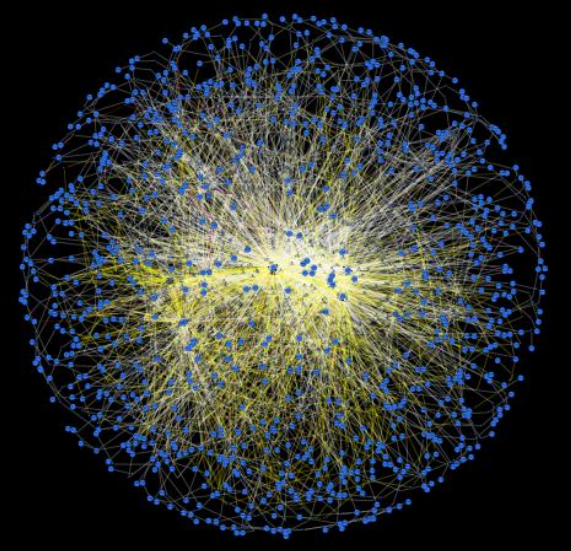
High-throughput experimental techniques (e.g., genome sequencing, RNA-seq and Tn-Seq) can rapidly identify and quantify cellular components, as well as their contributions to cellular fitness. However, even for well-studied organisms (e.g., Escherichia coli) we still do not completely understand the structure and activity of their metabolic and regulatory networks. Our group is interested in using computational approaches to generate hypotheses about microbial networks and to design experiments to test our understanding of these networks. By comparing model predictions to our experimental results we can identify missing or incorrect network connections (e.g., reactions, gene-to-reaction connections, and regulatory interactions). For example, by comparing model predictions with experimental growth measurements we have been able to find new metabolic functions and regulatory interactions in E. coli.
Funding:
- National Science Foundation
- U.S. Department of Energy
- Great Lakes Bioenergy Research Center
Related References:
- Kim J and JL Reed. Refining Metabolic Models and Accounting for Regulatory Effects. Current Opinion in Biotechnology. 29:34-38 (2014)
- Tervo CJ and JL Reed. BioMog: A Computational Framework for the De Novo Generation or Modification of Essential Biomass Components. PLOS One, 8(12):e81322 (2013).
- Tervo CJ and JL Reed. Experimental Design Tool for Systematizing Metabolic Discoveries and Model Development. Genome Biol, 13(12):R116 (2012).
- Barua D, et al. An automated phenotype-driven approach (GeneForce) for refining metabolic and regulatory models. PLOS Comp Biol, 6(10):e1000970 (2010).
- Reed JL, et al. Systems Approach to Refining Genome Annotation: Prediction and Validation of Gene Functions. PNAS. 103(46):17480-17484 (2006).
Metabolic Engineering
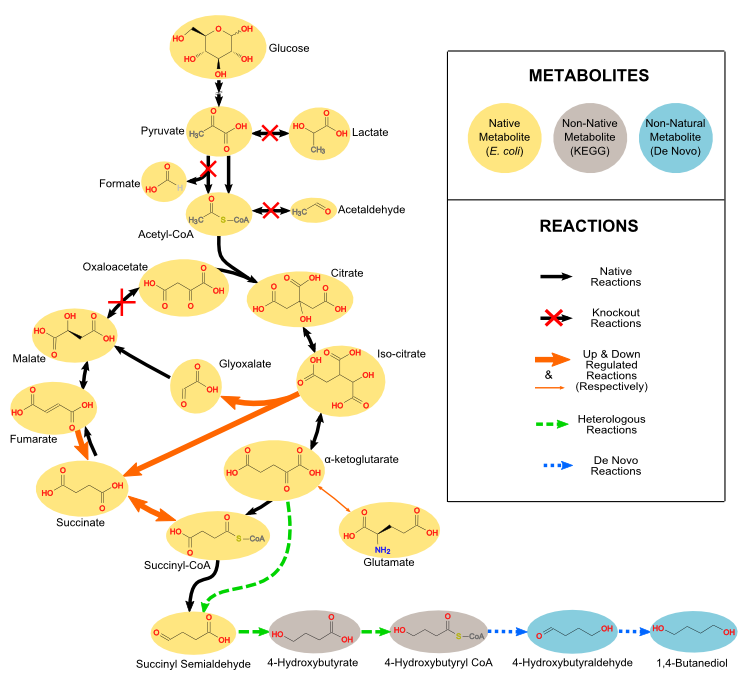
Microbes can be genetically engineered to produce useful chemicals (e.g., biofuels, pharmaceuticals, and commodity chemicals), but it is often difficult to determine which genetic changes are needed to improve chemical production. Our group is interested in developing computational approaches to predict how different genetic perturbations impact cellular production and growth rates. To accomplish this we measure metabolic fluxes (using 13C metabolic flux analysis) and build computational models that account for stoichiometric, thermodynamic, kinetic, and/or regulatory effects on metabolic fluxes. For example, we have developed approaches to design strains involving metabolic or regulatory gene deletions, reaction additions, and/or gene under- or over-expression. We are currently applying these methods to develop chemical production strains for a variety of microbes.
Funding:
- U.S. Department of Energy
- Great Lakes Bioenergy Research Center
- W.M. Keck Foundation
- SERDP
Related References:
- Tervo CJ and JL Reed. Expanding Metabolic Engineering Algorithms Using Feasible Space and Shadow Price Constraint Modules. Metabolic Engineering Communication, 1:1-11 (2014).
- Cotten C and JL Reed. Constraint-Based Strain Design Using Continuous Up and Downregulation of Fluxes. Biotechnology Journal, 8(5):595-604 (2013).
- Kim J and JL Reed. RELATCH: relative optimality in metabolic networks explains robust metabolic and regulatory responses to perturbations. Genome Biol, 13(9):R78 (2012).
- Kim J, et al. Large-Scale Bi-Level Strain Design Approaches and Mixed-Integer Programming Solution Methods. PLOS One, 6(9):e24162 (2011).
- Kim J, and JL Reed. OptORF: Optimal metabolic and regulatory perturbations for metabolic engineering of microbial strains. BMC Systems Biol, 4:53 (2010).
- Long MR, et al. Computational methods in metabolic engineering for strain design. Curr Opin Biotechnol, 34:135-141 (2015).
Microbial Interactions
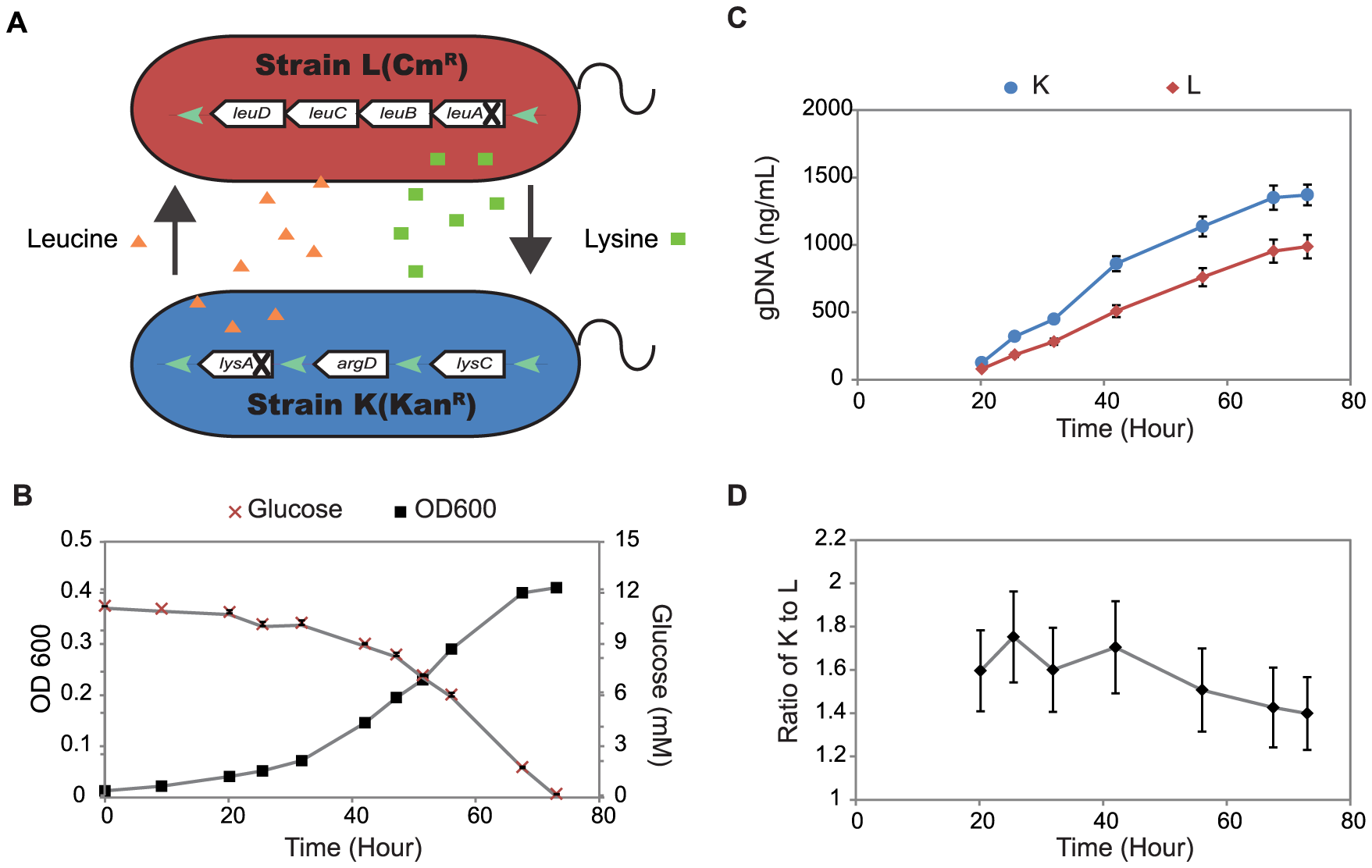
Microbes do not naturally live in isolation, but instead interact with other organisms in their environment. Many of these interactions are metabolic, where microbes exchange or compete for nutrients. Our group is interested in using systems biology approaches to study microbe-microbe and/or microbe-host interactions. We are working on developing models and approaches to predict metabolic fluxes and metabolic interactions in these complex systems. For example, we have used computational and experimental approaches to study a synthetic community of E. coli auxotrophs to hypothesize mechanisms for improved community fitness after adaptive evolution. In addition, we have analyzed Salmonella typhimurium proteomic datasets and virulence phenotypes to predict nutrients provided to S. typhimurium by host macrophages. We are currently building computational models to study which chemical cues are involved in the symbiotic relationship between Vibrio fischeri and its host, the Hawaiian bobtail squid.
Funding:
- Gordon and Betty Moore Foundation
- National Science Foundation
Related References:
- Zhang X and JL Reed. Adaptive Evolution of Synthetic Cooperating Communities Improves Growth Performance. PLOS One. 9(10):e108297 (2014).
- Raghunathan A, et al. Constraint-Based Analysis of Metabolic Capacity of Salmonella Typhimurium During Host-Pathogen Interaction. BMC Systems Biol, 3:38 (2009).
- Wier A, et al. Transcriptional patterns in both host and bacterium underlie a daily rhythm of ultrastructural and metabolic change in a beneficial symbiosis. PNAS, 107(5):2259-64 (2010).
- Hamilton JJ, et al. Thermodynamics and H2 Transfer in a Methanogenic, Syntrophic Community. PLOS Comp Biol. 11(7):e1004364 (2015).